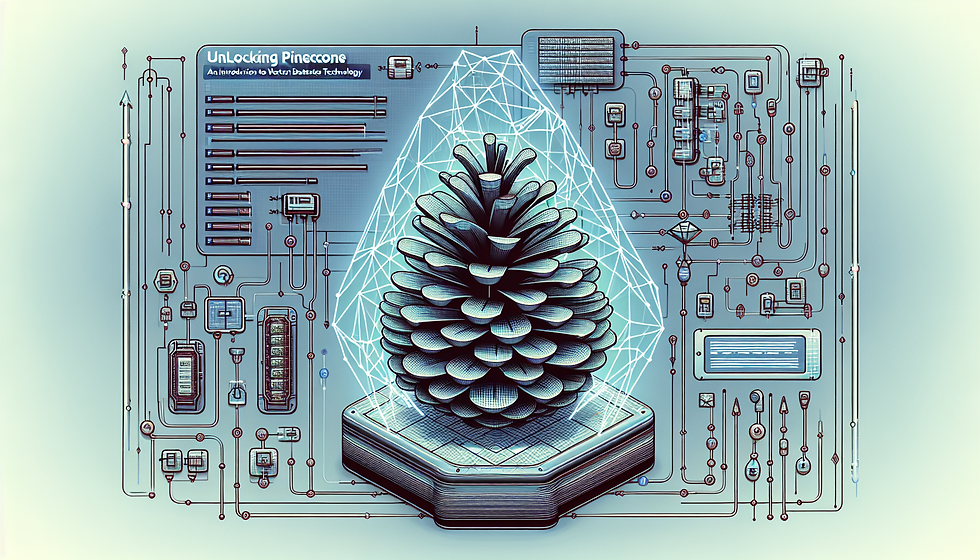
Understanding Vector Databases: A Primer
Vector databases represent an innovative approach to managing and querying data, and they are tailored specifically to handle vector embeddings that are commonly used in machine learning models. These embeddings, which are high-dimensional representations of data points, facilitate complex operations that are challenging for traditional database systems.
The Emergence of Pinecone
Pinecone is a fully managed vector database service that is engineered to simplify the storage, management, and retrieval of vector embeddings at scale. It allows developers and data scientists to easily build and deploy similarity search applications, enabling users to find related content, recommendations, or products based on their features.
Key Features of Vector Databases
Scalability: Vector databases are designed to scale effortlessly with large datasets, handling millions or even billions of vector embeddings without compromising performance.
Efficiency: They provide high-speed similarity search due to their ability to compress vector data and utilize indexing structures optimized for high-dimensional spaces.
Machine Learning Integration: Built to be ML-friendly, vector databases offer seamless integration with machine learning models, often supporting real-time embedding updates.
How Pinecone Works
Pinecone operates by indexing vector embeddings into a data structure that allows for efficient nearest neighbor searches. When a query vector is submitted, Pinecone rapidly returns the most similar vectors from the database. This capability is particularly valuable in domains such as natural language processing, computer vision, and user personalization.
Use Cases for Vector Databases
Vector databases shine in a variety of innovative applications. Here are several scenarios where services like Pinecone are invaluable:
Personalized Recommendations: They can deliver content or product recommendations that are tailored to the user’s preferences based on similarity matching.
Semantic Search: By understanding the context of search queries, vector databases facilitate richer and more relevant search results.
Fraud Detection: They can identify patterns and anomalies in transaction data to signal potential fraudulent activity more effectively than traditional databases.
Challenges and Considerations
While vector databases offer a host of benefits, they also present unique challenges:
Data Preparation: Vector embeddings need to be prepared and maintained, which can be a complex task depending on the size and dynamism of the dataset.
Infrastructure: The high-dimensional nature of vector data demands substantial computational resources, making infrastructure management crucial for optimal performance.
Learning Curve: The adoption of vector databases may require upskilling or new hires, as there can be a learning curve associated with understanding and leveraging this technology.
The Future of Vector Database Technology
As machine learning and artificial intelligence continue to evolve, the importance and relevance of vector databases similarly escalate. Platforms like Pinecone are at the forefront of this technological shift, providing accessible, scalable, and efficient systems for complex data-driven applications. With the continuous growth in the amount of data generated, and the increasing reliance on ML models, vector databases are poised to become an integral part of the data management landscape.
In conclusion, by unlocking the potential of vector databases through services like Pinecone, organizations can harness the full power of their data to drive innovation, streamline processes, and create more personalized user experiences. As this technology ecosystem matures, we can expect additional advancements that will further revolutionize the way we handle and analyze complex datasets.
Comments